A technical paper was accepted on IEEE Transactions on Visualization and Computer Graphics
CMIC present a method to reconstruct real-world environmental lighting, encoded as a reflection map (RM), from a conventional photograph.
CMIC post-doc researcher Andrew Chalmers, Junhong Zhao, Daniel Medeiros and associate professor Taehyun Rhee developed a new method to reconstruct real-world environmental lighting.
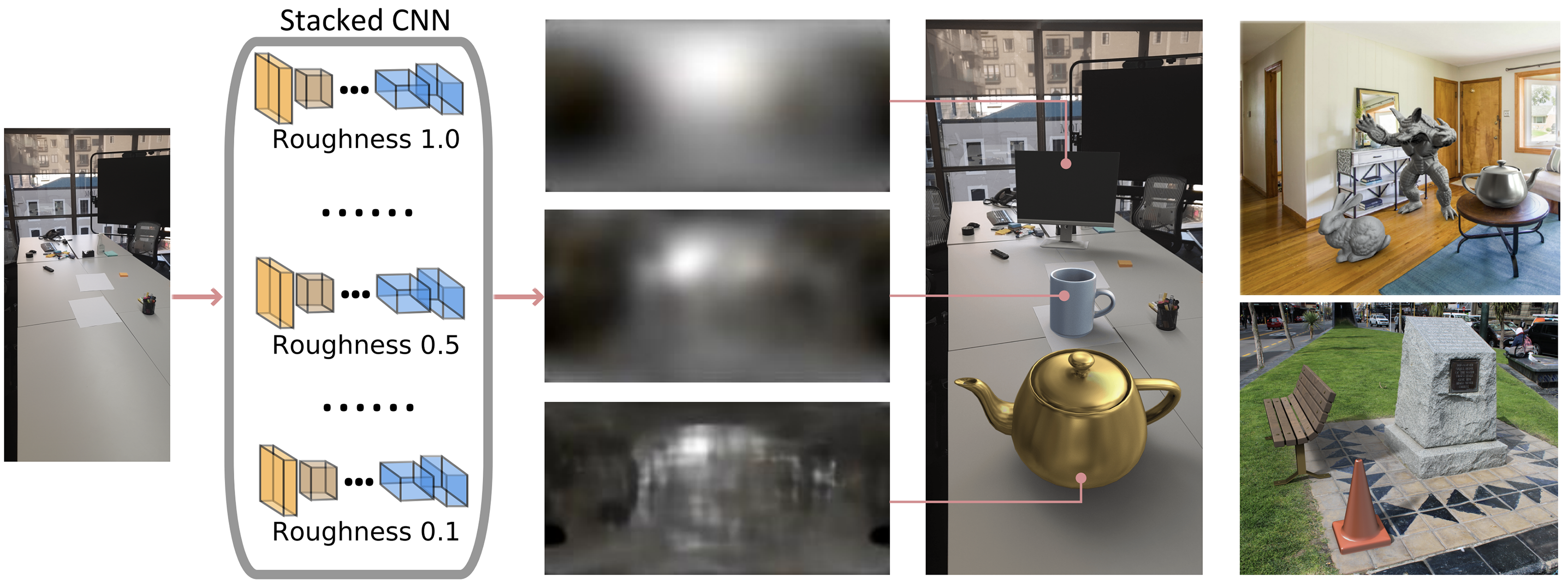
Corresponding lighting and reflectance between real and virtual objects is important for spatial presence in augmented and mixed reality (AR and MR) applications. We present a method to reconstruct real-world environmental lighting, encoded as a reflection map (RM), from a conventional photograph. To achieve this, we propose a stacked convolutional neural network (SCNN) that predicts high dynamic range (HDR) 360° RMs with varying roughness from a limited field of view, low dynamic range photograph. The SCNN is progressively trained from high to low roughness to predict RMs at varying roughness levels, where each roughness level corresponds to a virtual object's roughness (from diffuse to glossy) for rendering. The predicted RM provides high-fidelity rendering of virtual objects to match with the background photograph. We illustrate the use of our method with indoor and outdoor scenes trained on separate indoor/outdoor SCNNs showing plausible rendering and composition of virtual objects in AR/MR. We show that our method has improved quality over previous methods with a comparative user study and error metrics.
- "Reconstructing Reflection Maps using a Stacked-CNN for Mixed Reality Rendering",
IEEE Transactions on Visualization and Computer Graphics
Andrew Chalmers (CMIC, Victoria University of Wellington)
Junhong Zhao (CMIC, Victoria University of Wellington)
Daniel Medeiros (CMIC, Victoria University of Wellington)
Taehyun Rhee (Victoria University of Wellington)